
Magnovate's
Artificial Intelligence
A transit system that learns from experience
Magnovate’s flexible network architecture and packet switching operating model allows the use of artificial intelligence to optimize vehicle performance and network efficiency. Our breakthrough AI technology learns traffic patterns and uses a set of parameters to assign the fastest route to each vehicle so that they’re sent to the right place at the right time using the most efficient path.
How can AI improve rapid transit?
Low capacity transit at low cost … or high capacity transit at high cost? Choose one. This is the tradeoff decision that transit planners and rail owners face.
High capacity transit modes like subways and commuter trains can move around 40,000 passengers per hour. They cost $300-$600 million per mile because they rely on 300 ton trains sets that require heavy, expensive infrastructure. Trains have poor utilization because of their inflexible operating model. Tracks remain empty 99% of the time and they don’t have the ability to adapt to fluctuations in demand. During periods of peak demand trains are often overcrowded and during non-peak hours trains often run almost empty – wasting enormous amounts of energy.
In contrast, lightweight systems like autonomous transit networks (ATNs) can move around 4,000 passengers per hour at a cost of $15 million per mile. ATNs are cheap to build because they use fleets of lightweight pods that only weigh around 1 ton each. The advantage of ATNs compared with conventional transit is that they offer highly flexible on-demand point-to-point service but low speeds and low capacity limits their utility.
In order for cities to realize the congestion relief and environmental benefits that rapid transit offers the tradeoff problem must be solved. Flexible hardware and AI can help.
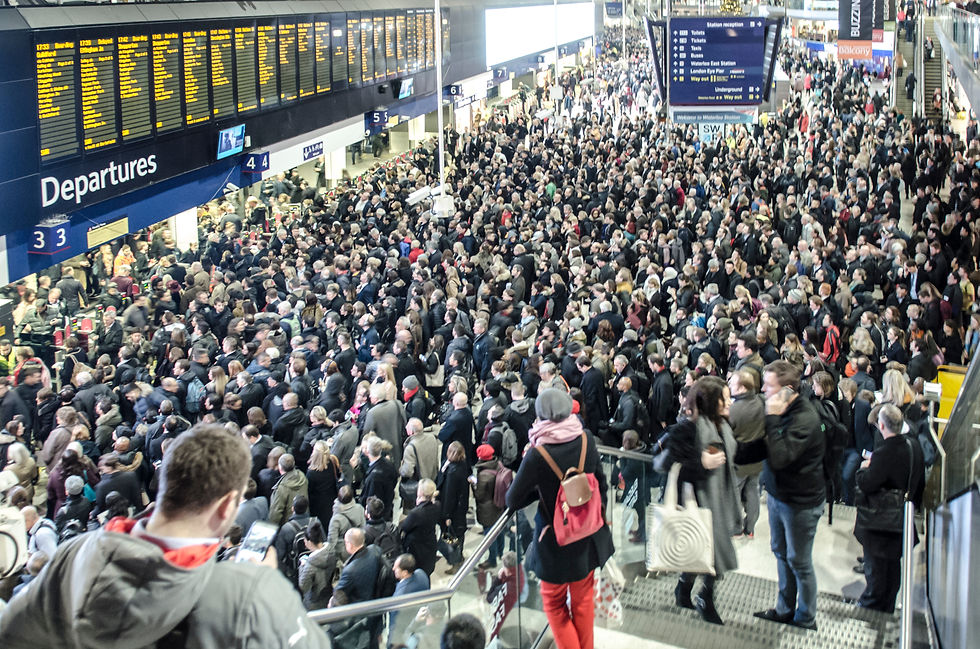
How does Magnovate apply AI?
Magnovate's AI enabled control system works by intelligently routing vehicles based on algorithms that choose the fastest and most efficient route for each trip. This enables very high capacity with continuous flows of lightweight vehicles.
Taken together with high speeds, short vehicle headways and non-stop routing, the optimization of Magnovate networks with AI translates into a breakthrough in how a transit system delivers value by eliminating the tradeoffs between passenger throughput, cost, service frequency, energy consumption and the quality of service that passengers receive.
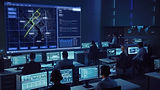
Intelligent Empty Vehicle Management
A critical function for achieving high throughput with large fleets of autonomous vehicles is intelligent empty vehicle management (EVM). Magnovate’s AI enabled EVM system uses machine learning to optimize throughput by learning traffic patterns so that vehicles are sent to the right place at the right time.
Most of the proposed EVM solutions are based on algorithms designed by human programmers that are focused on the optimal reallocation of empty vehicles based on a central data base in which historical demand and actual positions of empty vehicles are stored. Our AI technology is based on a much larger and more comprehensive set of parameters such as measuring physical features of the network, historical data, and current traffic conditions, to name a few. A number of weights and thresholds are applied to these features to inform the decisions on where to send empty vehicles.
The EVM system uses decentralized data on real-time demand, past demand, vehicles at stations and on the move (performing full and empty trips). Every station on the network retrieves data from its neighboring stations, which allows for distributed implementation. If demand follows historical trends, the AI platform uses predictive algorithms to distribute vehicles. It can also recognize non-typical demand, such as a large sporting event, and inform the EVM system to cover this unusual demand. It’s this ability to optimize each vehicle’s routing to align with demand that makes deep learning so powerful.
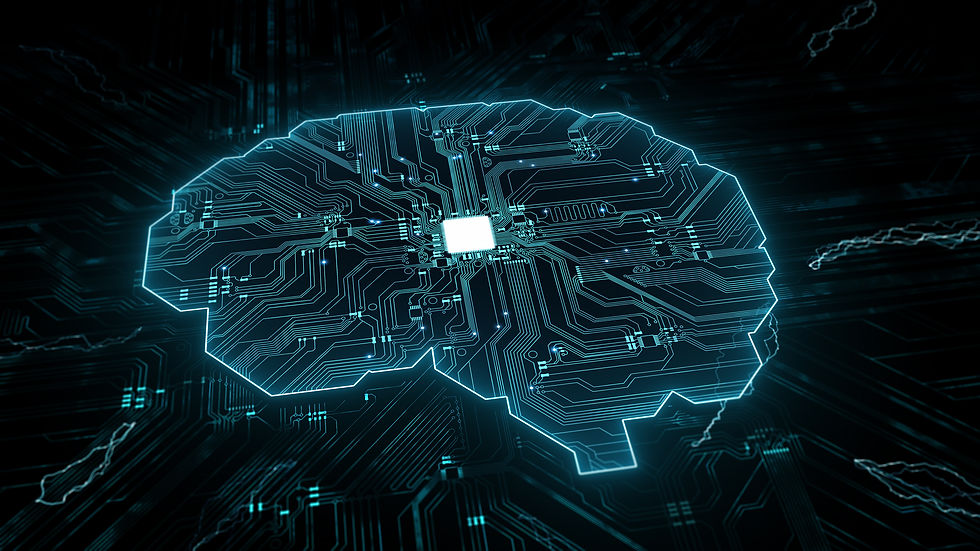